Accelerating SLE Trials: Early Derived Endpoint Reduces Sample Size & Enhances Trial Efficiency
Key Takeaway
ML early derived endpoints can help reduce trial timelines, optimize resource allocation, and increase the probability of success in complex diseases like SLE
Challenge
Clinical trials in Systemic Lupus Erythematosus (SLE) are inherently challenging due to disease heterogeneity and the extended follow-up periods required for common endpoints. While adaptive trials—adjusting treatment arms based on accumulated trial data—can offer some flexibility, the long time to primary endpoints often limits trial efficiency and decision-making
Solutions
- PhaseV analyzed a Phase II randomized controlled trial (RCT) for a lupus drug, where the primary endpoint was BILAG score improvement at 24 weeks.
- PhaseV’s ML-based analysis identified BILAG data at week 8 as a reliable early derived endpoint to predict response at week 24, enabling trial adaptation
Proven Results
PhaseV designed and simulated an adaptive trial using the early derived endpoint, demonstrating a significant reduction in required sample size and an increase in overall trial efficiency
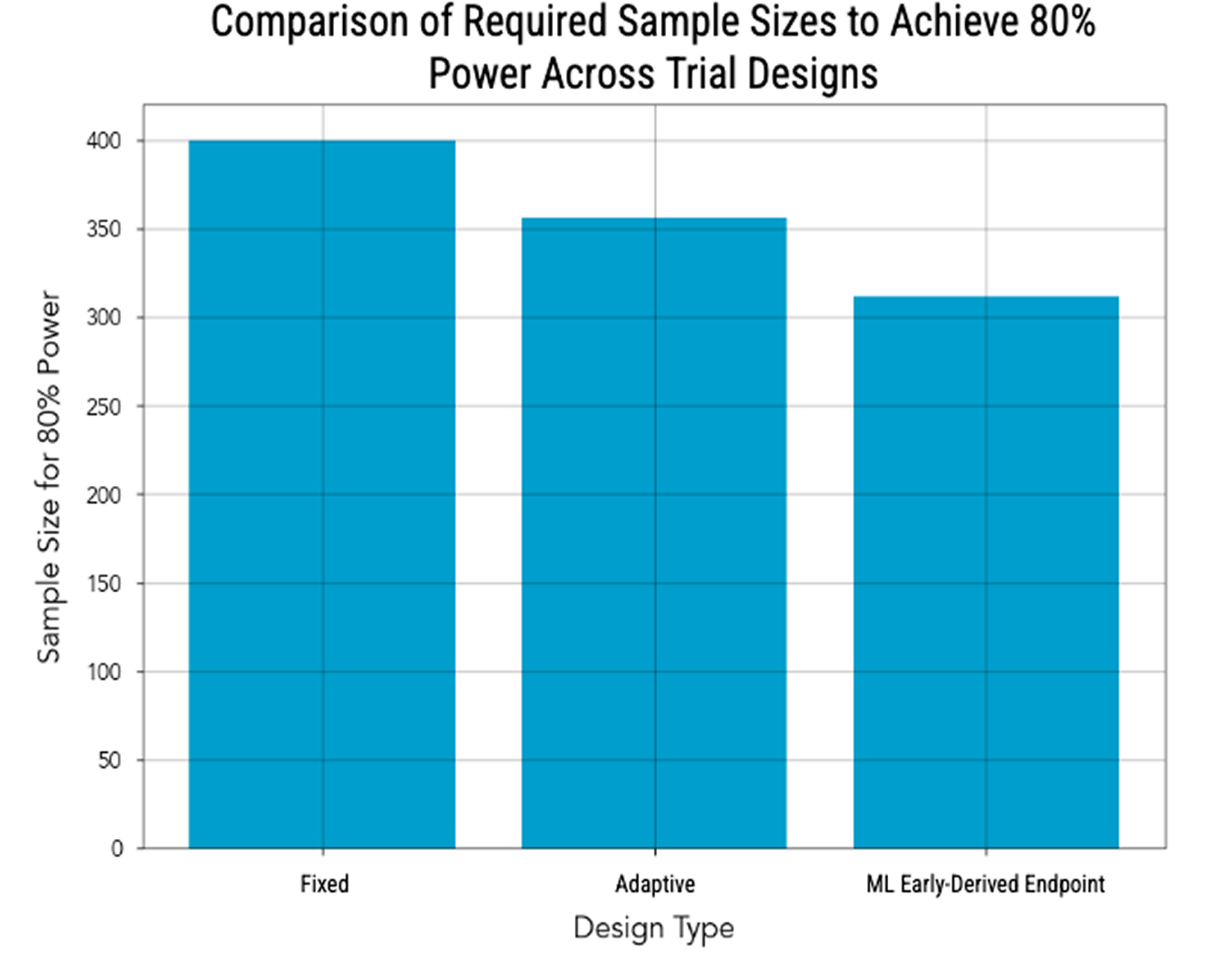
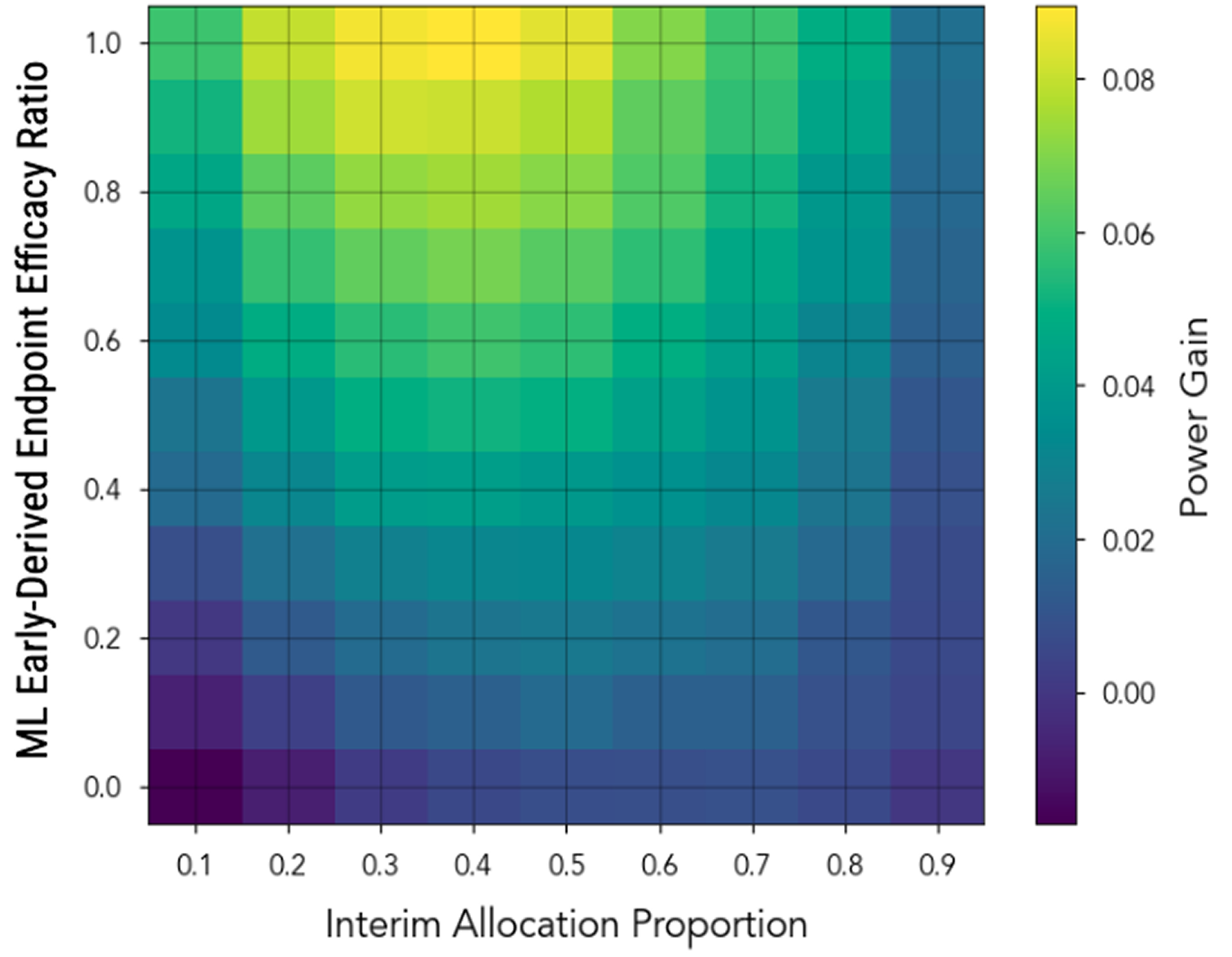
Real-World Impact
By leveraging early response signals, the trial design optimized patient selection and accelerated decision-making, allowing for a more efficient and cost-effective path toward Phase III