Advancing Oncology Trials with Covariate Adjustment
Abstract
In the dynamic field of oncology, clinical trials’ accuracy and efficiency are crucial. Patient heterogeneity—characterized by diverse factors such as age, genetics, and baseline disease status—complicates the interpretation of trial results. Addressing this variability is essential for obtaining clear and reliable insights into treatment efficacy.
Covariate adjustment is an advanced statistical technique designed to account for baseline differences among patients. By incorporating key covariates into the analysis of results, we refine the accuracy of treatment effect estimates and accelerate the trial process, paving the way for more reliable and quicker access to life-saving therapies.
PhaseV and iOMEDICO are incorporating vast data with advanced causal inference to ensure efficient covariate selection based on high-quality curated data and deep experience. This advanced approach directly enhances oncology trial design, optimizes data efficiency, and improves trial outcomes.
Introduction: Challenges in Oncology RCTs
Oncology clinical trials are essential for developing new cancer therapies and improving patient outcomes. However, these trials face numerous challenges that can impede their progress and effectiveness.
Heterogeneity in oncology is a significant challenge, with high genetic, molecular, and phenotypic diversity among different cancer types and within tumors of the same type. This variability impacts disease progression, clinical presentation, and treatment responses, posing significant challenges in developing and assessing new therapies. In terms of clinical trials, this diversity can obscure the true efficacy of a treatment and complicate the interpretation of trials.
Securing adequate sample size and statistical power in oncology trials is challenging, especially for rare malignancies or specific subgroups. Long follow-up periods for time-to-event endpoints, like overall survival, lead to patient dropouts and increased censoring, compromising the study's power. In addition, the ongoing development of new therapies and combination treatments leads to numerous simultaneous trials for the same indications, increasing competition for patient recruitment and further compromising achievable sample sizes.
In conclusion, the pronounced heterogeneity of oncological diseases and the difficulties in attaining adequate sample sizes and statistical power present major obstacles for clinical trials seeking to demonstrate the efficacy of novel treatments. Overcoming these challenges necessitates the implementation of innovative trial designs and advanced methods for analyzing trial outcomes.
The Benefits of Covariate Adjustment
Covariate adjustment is a statistical technique used to account for variables that might affect the outcome of a study, thus isolating the true effect of the treatment being evaluated. In oncology trials, these variables—known as prognostic factors—might include factors like disease stage, performance status, or genetic markers.
Let’s consider, as an example, a clinical trial that compares the effectiveness of a new cancer drug, Drug A, to a standard treatment, Drug B, in patients with melanoma. The primary outcome is overall survival (OS). It is known that the presence of certain genetic mutations affects patient survival. If Drug A is given by chance to a group of patients containing more patients with mutations associated with poor prognosis, the drug’s effect might appear weaker due to confounding. Adjusting for genetic mutations in the analysis accounts for the difference between the treatment groups, thus overcoming this challenge. It’s important to note that stratified randomization, while useful in general, is not easily applicable for genetic mutations due to the complexity and variability of these markers. As a result, adjustment in the analysis becomes important to accurately assess the drug’s efficacy.
More importantly, since patient survival is influenced by the presence of genetic mutations, some of the variability in observed survival times is inherently due to the genetic mutations rather than the treatment itself. Even if the treatment groups were perfectly balanced, accounting for the prognostic component in observed survival times reduces the overall variability of the outcome and improves the accuracy of treatment effect estimation. Generally speaking, incorporating important prognostic factors into the analysis helps to better account for the variability in the outcome variable. By effectively removing the part of this variability attributed to prognostic factors, the outcome distributions of the different arms become more clearly separated. This results in increased statistical power and a more precise estimation of the treatment effect.
Selecting the appropriate prognostic factors for analysis can be challenging. This task requires a solid clinical foundation and may also involve advanced data-driven techniques for building prognostic models and feature selection. However, when applied correctly, covariate adjustment can substantially enhance statistical power and also address potential imbalances between treatment groups, ensuring comparability across different cohorts. This increases the likelihood of successfully detecting true treatment effects. Additionally, if a negative result is obtained (i.e. no significant treatment effect), covariate adjustment provides greater confidence in the validity of that result. The combined expertise of iOMEDICO in oncology data and PhaseV in advanced data analysis for oncological clinical trials creates a powerful synergy, enabling robust and effective covariate adjustment that can significantly improve trial outcomes.
.png)
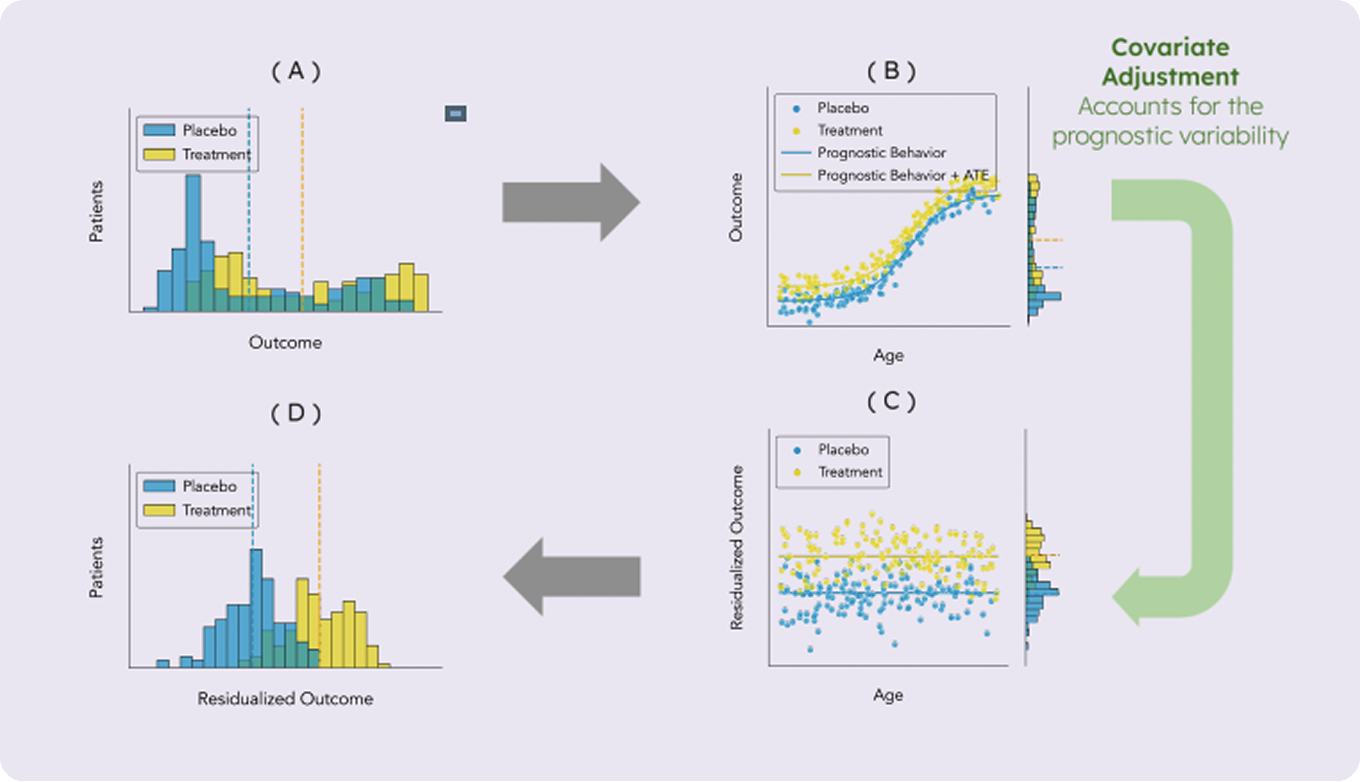
Covariate adjustment is endorsed by regulatory agencies such as the FDA and EMA, as it is generally robust and is well aligned with established clinical practices. Extensive research supports this approach, demonstrating that it enhances the accuracy of treatment effect estimation and increases the trial’s power without compromising the integrity of the results. In recent years, advanced methods for covariate adjustment have been developed, expanding beyond the traditional Analysis of Covariance (ANCOVA) approach and providing novel ways to enhance treatment effect estimation.
Case Study: The AVAGLIO Trial in Glioblastoma Multiforme
The AVAGLIO trial was a phase III clinical trial investigating the efficacy and safety of bevacizumab (Avastin) in combination with radiotherapy and temozolomide in patients with newly diagnosed Glioblastoma, a highly aggressive brain tumor. The primary goal of the AVAGLIO trial was to determine if adding bevacizumab to the standard treatment regimen of radiotherapy and temozolomide would improve overall survival (OS) and progression-free survival (PFS).
A total of 921 patients with newly diagnosed Glioblastoma were allocated to receive either bevacizumab plus radiotherapy and temozolomide (experimental arm, 458 patients), or placebo plus radiotherapy and temozolomide (control arm, 463 patients).
The AVAGLIO trial demonstrated that adding bevacizumab to the standard treatment regimen for newly diagnosed Glioblastoma significantly improved PFS (10.6 vs. 6.2 months median PFS with bevacizumab versus placebo; hazard ratio 0.64), but did not extend OS.
A previously published retrospective analysis of the AVAGLIO trial data suggested potential OS benefit within a specified subgroup of patients with proneural Glioblastoma.
PhaseV conducted an alternative analysis that employed a much smaller set of covariates for adjustment and also considered the full (biomarker-evaluable) study population. We used both unadjusted and covariate-adjusted models to demonstrate the benefit of adjusting for prognostic covariates in the analysis.
Analysis 1: Full Study Population
The unadjusted Cox proportional hazards (PH) model only included a single term, representing the treatment. This model yielded a non-significant average treatment effect (ATE) with a hazard ratio (HR) of 0.88 (95% CI, 0.70 to 1.12) and a p-value of 0.314. In other words, based on this simple regression model, we cannot conclude that a treatment effect exists.
By leveraging covariate adjustment, we can conduct a much more powerful analysis. In this instance, we concentrated on three key prognostic factors: age at diagnosis, Karnofsky Performance Status (KPS), and MGMT methylation status. These factors are well-established in their association with Glioblastoma prognosis and are therefore highly relevant for covariate adjustment.
The adjusted Cox PH model incorporated the treatment term along with additional terms for the three prognostic factors. This model yielded an ATE estimate with an HR of 0.79 (95% CI, 0.62, 1.00) and a p-value of 0.0516. Although the p-value remains just above the conventional 0.05 significance level, the enhanced statistical power provided by covariate adjustment reveals a more pronounced effect estimate than in the unadjusted model. This is also manifested in an improved z-score of -1.95 vs. an initial -1.01. Consequently, this model offers evidence of an overall survival benefit for a much broader patient population than previously reported.
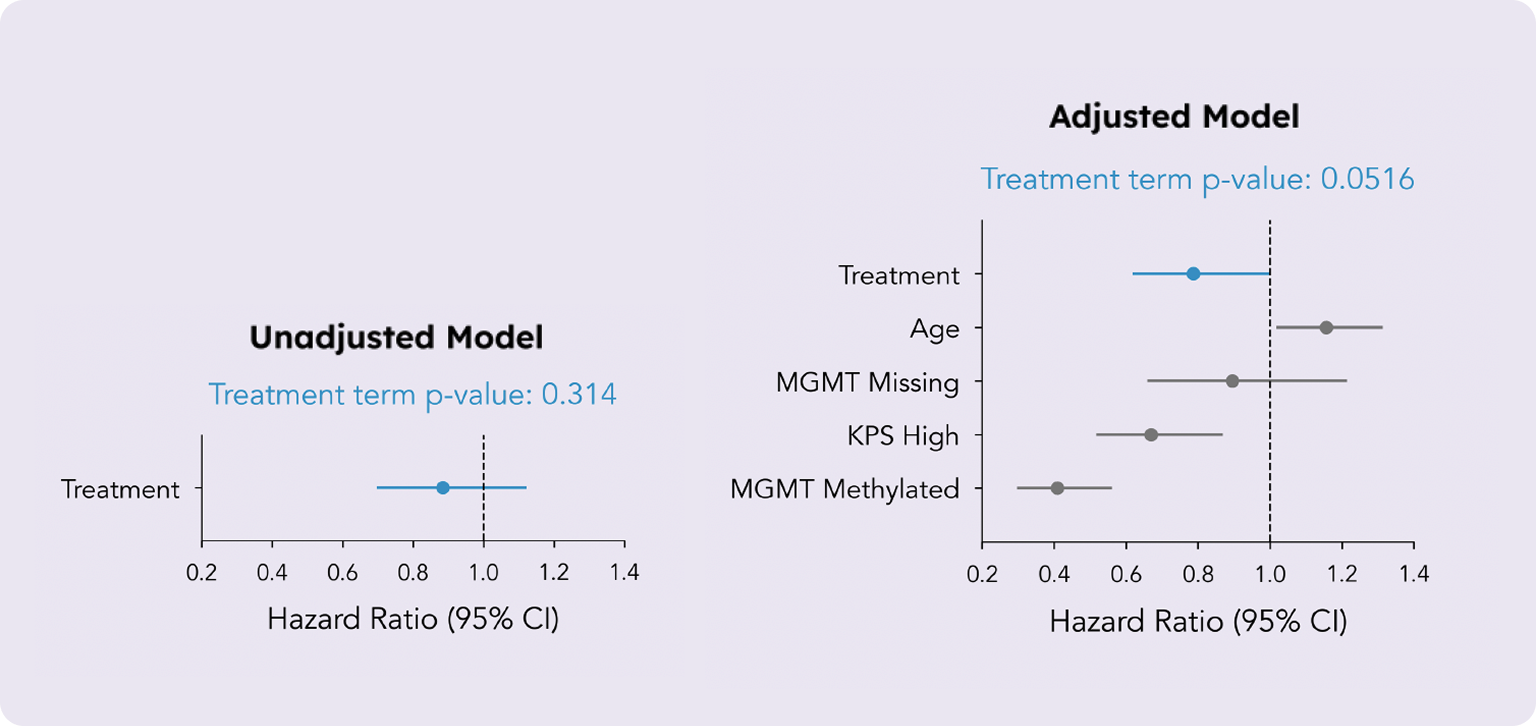
The unadjusted model produced a non-significant treatment effect estimate (top). However, by incorporating just three well-established prognostic factors, the treatment effect estimate was substantially improved, providing evidence of an overall survival benefit (bottom).
Analysis 2: Patients with Proneural Glioblastoma
We applied the same analytical approach to the subgroup of patients with proneural Glioblastoma (n = 112), and the benefits of covariate adjustment were even more pronounced. This time, the unadjusted model estimated an ATE with a hazard ratio (HR) of 0.67 (95% CI, 0.44 to 1.04) and a p-value of 0.0742. While this result suggests some evidence of an effect, it does not reach the standard significance threshold of 0.05.
In this analysis, we included an additional covariate for WHO Performance Status, another known prognostic factor for Glioblastoma, alongside age, KPS, and MGMT methylation status. The adjusted model yielded an estimated ATE with a hazard ratio (HR) of 0.46 (95% CI, 0.28 to 0.77) and a p-value of 0.0029. This clearly significant result further highlights the substantial advantages of covariate adjustment in refining treatment effect estimates.
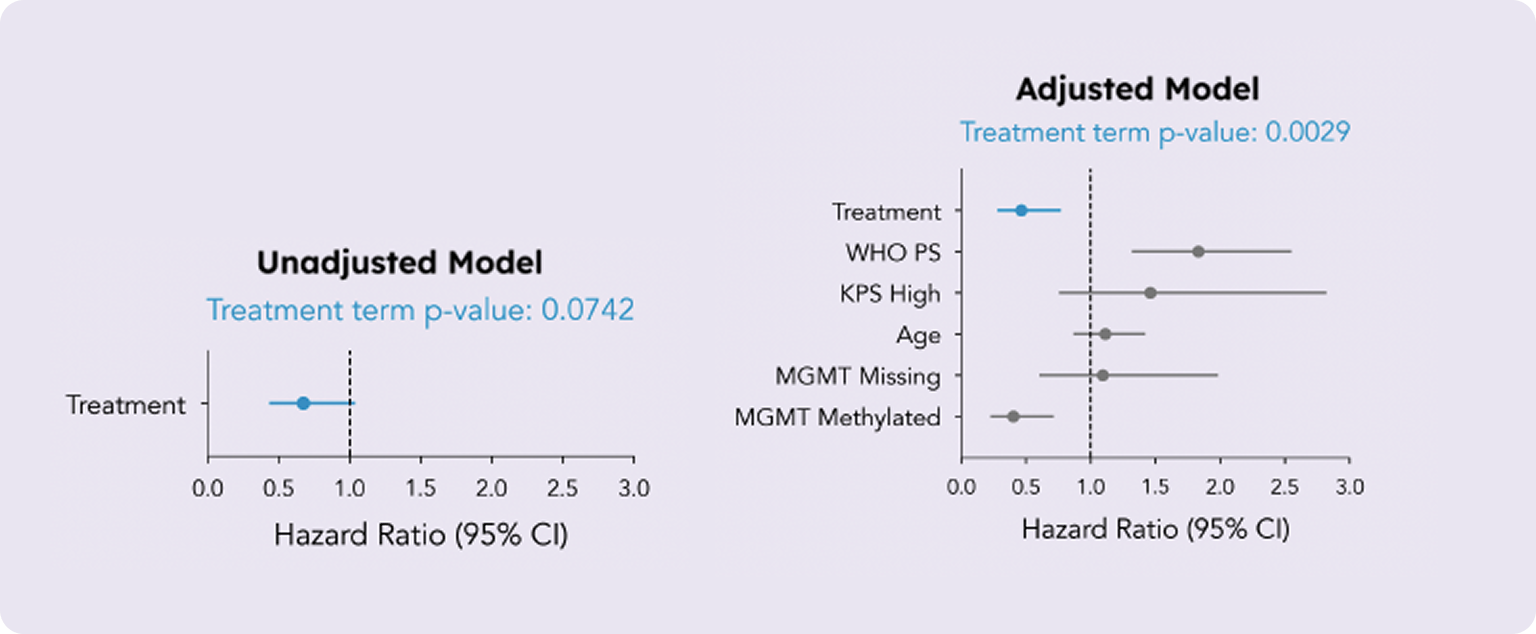
Leveraging RWD to Enhance RCTs
Real-world data (RWD) refers to health-related data collected from various sources outside of traditional clinical trials. These sources may include Electronic Health Records (EHRs), data from insurance claims/billing records, patient registries, and patient-reported outcomes.
RWD is much larger in scope and diverse in comparison to clinical trials data, and better represents the general population. Thus, it can help bridge the gap between the controlled conditions of clinical trials and everyday clinical practice. RWD provides comprehensive information about patient demographics, disease characteristics, comorbidities, and prior treatments. The wealth of information in RWD, combined with existing clinical knowledge, can help develop more robust and reliable prognostic models. This approach improves the ability to reduce variability in RCT datasets and enhances the probability of success.
iOMEDICO maintains extensive real-world databases with prospectively collected, long-term documentation of over 45,000 cancer patients. The data is differentiated from other datasets as it includes patient-reported outcome data as well as longitudinal clinical and biomarker data, thus making its integration into clinical design and analysis simpler and more direct. The cutting-edge ML-based methods by PhaseV, when combined with the comprehensive dataset maintained by iOMEDICO, hold unique promise for the important task of covariate adjustment in oncology studies.
The Efficient Choice for Oncology Trials
Covariate adjustment is a widely accepted method with well-documented advantages. It enables more accurate and unbiased treatment effect estimates. Additionally, it increases statistical power by accounting for the prognostic variability present in the data. RWD can enhance covariate adjustment by offering a richer and more varied dataset, which facilitates the precise identification of relevant prognostic factors and a deeper understanding of their effects on treatment outcomes.
This way, we can substantially improve clinical trial efficiency. Given the challenges in oncology research, these efficiency gains could be pivotal in determining the success or failure of a trial and are crucial for achieving more reliable and broadly applicable results.
References
- U.S. Food and Drug Administration. Adjusting for Covariates in Randomized Clinical Trials for Drugs and Biological Products: Guidance for Industry. U.S. Food and Drug Administration. May 2023.
- European Medicines Agency. Guideline on Adjustment for Baseline Covariates in Clinical Trials. European Medicines Agency. February 2015.
- Kahan BC, Jairath V, Doré CJ, et al. The risks and rewards of covariate adjustment in randomized trials: an assessment of 12 outcomes from 8 studies. Trials. 2014;15:139. doi:10.1186/1745-6215-15-139.
- Holmberg MJ, Andersen LW. Adjustment for baseline characteristics in randomized clinical trials. JAMA. 2022;328(21):2155-2156. doi:10.1001/jama.2022.21506.
- Harrell F. Incorrect Covariate Adjustment May Be More Correct than Adjusted Marginal Estimates. Published June 29, 2021. Accessed from https://www.fharrell.com/post/robcov/. Vanderbilt University School of Medicine Department of Biostatistics.
- Morris TP, Walker AS, Williamson EJ, White IR. Planning a method for covariate adjustment in individually randomised trials: a practical guide. Trials. 2022;23(1):328. doi:10.1186/s13063-022-06097-z.
- Van Lancker K, Bretz F, Dukes O. Covariate adjustment in randomized controlled trials: general concepts and practical considerations. Clin Trials. 2024;0(0). doi:10.1177/17407745241251568.
- Li Y, Sabbaghi A, Walsh JR, Fisher CK. Prognostic covariate adjustment for logistic regression in randomized controlled trials. arXiv. 2024. Available at: https://arxiv.org/abs/2402.18900.
- Schuler A, Walsh D, Hall D, Walsh J, Fisher C. Increasing the eiciency of randomized trial estimates via linear adjustment for a prognostic score. Int J Biostat. 2021;18(2):329-356. doi:10.1515/ijb-2021-0072.
- Chinot OL, Wick W, Mason W, et al. Bevacizumab plus radiotherapy–temozolomide for newly diagnosed glioblastoma. N Engl J Med. 2014;370:709-722. doi:10.1056/NEJMoa1308345.
- Sandmann T, Bourgon R, Garcia J, Li C, Cloughesy T, Chinot OL, Wick W, et al. Patients with proneural glioblastoma may derive overall survival benefit from the addition of bevacizumab to first-line radiotherapy and temozolomide: retrospective analysis of the AVAglio trial. J Clin Oncol. 2015;33(25):2735-2744. doi:10.1200/JCO.2015.61.5005."